The Lab Story
The Laboratory for Active and Attentive Vision (LAAV) has its roots in the original Computer Vision Laboratory at the University of Toronto founded by John Tsotsos in 1980. In those years it was part of the Artificial Intelligence Group in the Department of Computer Science. There, Tsotsos also founded the Technical Report Series: Research in Biological and Computational Vision (1984 – 1996). In January 2000, Tsotsos moved to York University to take up the Directorship of the Centre for Vision Research and a portion of that lab followed him. The history of the current lab thus goes back to 1980 and includes a significant number of students, post-docs and publications from the pre-York era.
At York, the Laboratory for Active and Attentive Vision is situated within the Department of Electrical Engineering and Computer Science in the Lassonde Scholl of Engineering. It is also one of the many labs in the much larger Centre for Vision Research (cvr.yorku.ca) and in the new Centre for Innovation in Compuoting at Lassonde (http://ical.lassonde.yorku.ca/). With a rich set of international collaborators, and a well-equipped infrastructure the lab is an exciting research focus for interdisciplinary research on a variety of vision topics within seven themes: Computational Neuroscience, Vision, Visually Guided Robotics, Computer Vision, Visual Attention, Cognitive Architectures and Human Vision and Visual Behaviour. The Venn diagram below shows the breadth of projects within these themes.
Research Areas
Click on subtopics to view current active projects.
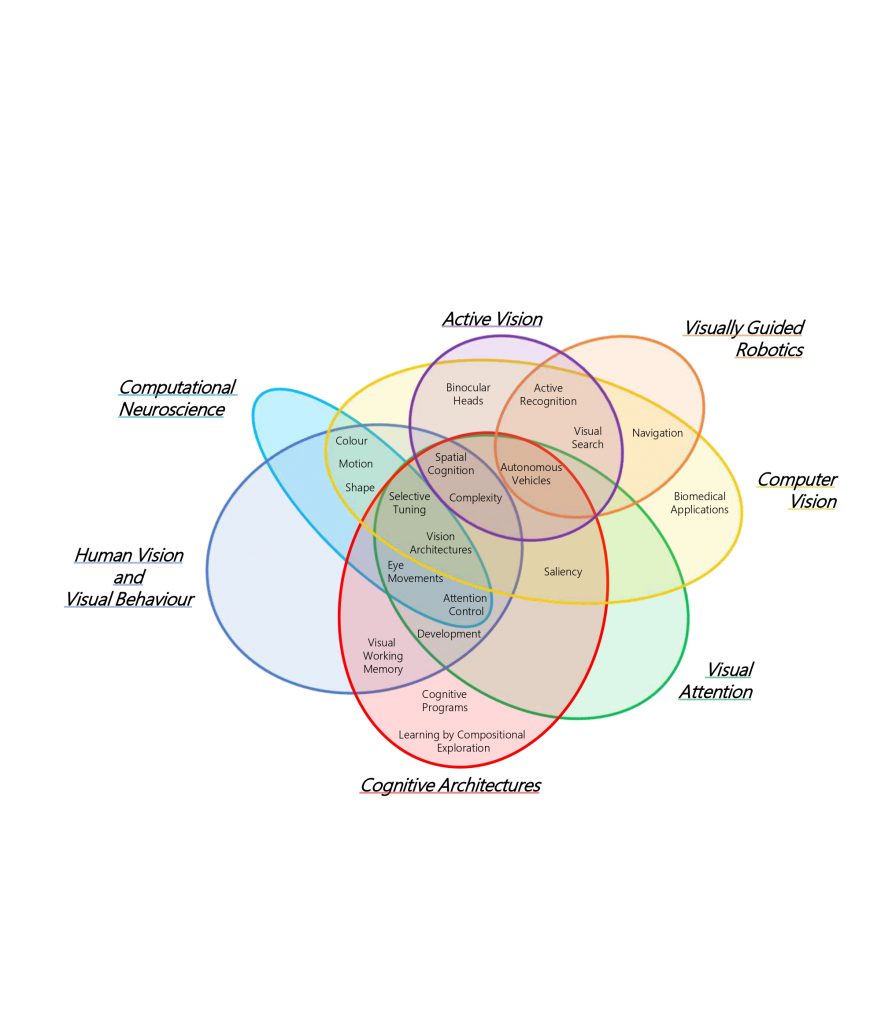