Shape
The Roles of Endstopped and Curvature Tuned Computations in a Hierarchical Representation of 2D Shape
That shape is important for perception has been known for almost a thousand years (thanks to Alhazen in 1083) and has been a subject of study ever since by scientists and philosophers (such as Descartes, Helmholtz or the Gestalt psychologists). Shapes are important object descriptors. If there was any remote doubt regarding the importance of shape, recent experiments have shown that intermediate areas of primate visual cortex such as V2, V4 and TEO are involved in analyzing shape features such as corners and curvatures. The primate brain appears to perform a wide variety of complex tasks by means of simple operations. These operations are applied across several layers of neurons, representing increasingly complex, abstract intermediate processing stages. Recently, new models have attempted to emulate the human visual system. However, the role of intermediate representations in the visual cortex and their importance have not been adequately studied in computational modeling. This paper proposes a model of shape-selective neurons whose shape-selectivity is achieved through intermediate layers of visual representation not previously fully explored. We hypothesize that hypercomplex – also known as endstopped – neurons play a critical role to achieve shape selectivity and show how shape-selective neurons may be modeled by integrating endstopping and curvature computations. This model – a representational and computational system for the detection of 2-dimensional object silhouettes that we term 2DSIL – provides a highly accurate fit with neural data and replicates responses from neurons in area V4 with an average of 83% accuracy. We successfully test a biologically plausible hypothesis on how to connect early representations based on Gabor or Difference of Gaussian filters and later representations closer to object categories without the need of a learning phase as in most recent models.
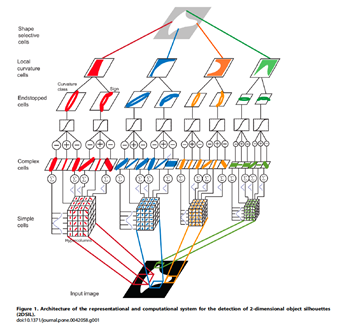
Publications
Rodrıguez-Sanchez AJ, Tsotsos JK (2012) The Roles of Endstopped and Curvature Tuned Computations in a Hierarchical Representation of 2D Shape. PLoS ONE 7(8): e42058. doi:10.1371/journal.pone.0042058
Recurrent Border Ownership Assignment
The face-vase illusion introduced by Rubin demonstrates how one can switch between two different interpretations depending on how the figure outlines are assigned. This border ownership assignment is an important step in the perception of forms. In this work, we introduce a biologically plausible model for border ownership assignment called the Recurrent Border Ownership (RBO) model. Our proposed model incorporates early recurrence from the dorsal pathway as well as ventral lateral modulations, combining global and local context. While RBO meets known biological properties of the relevant brain regions, a computer vision scientist can view our computational model as a convolutional neural network (CNN) with certain constraints. Our simulation results show that our model border ownership neurons, similar to their biological counterparts, exhibit difference of responses to figures on either side of the border.
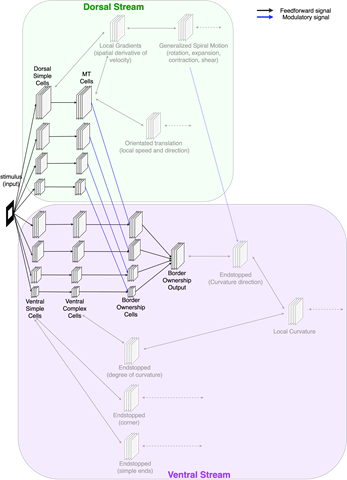
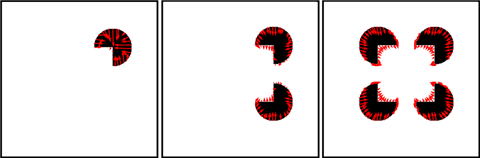
Publication
Mehrani, P., & Tsotsos, J. K. (2019). Early recurrence enables figure border ownership. arXiv preprint arXiv:1901.03201.