Calden will present “Spatially Binned ROC: A Comprehensive Saliency Metric” at CVPR 2016
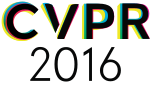
Authors: Calden Wloka and John Tsotsos
We argue that it is more informative to directly measure the effect of spatial bias on algorithm performance rather than try to correct for it. To capture and quantify these known sources of bias, we propose a novel metric for measuring saliency algorithm performance: the spatially binned ROC (spROC). This metric provides direct insight into the spatial biases of a saliency algorithm without sacrificing the intuitive raw performance evaluation of traditional ROC measurements. By quantitatively measuring the bias in saliency algorithms, researchers will be better equipped to select and optimize the most appropriate algorithm for a given task. We use a baseline measure of inherent algorithm bias to show that Adaptive Whitening Saliency (AWS), Attention by Information Maximization (AIM), and Dynamic Visual Attention (DVA) provide the least spatially biased results, suiting them for tasks in which there is no information about the underlying spatial bias of the stimuli, whereas algorithms such as Graph Based Visual Saliency (GBVS) and Context-Aware Saliency (CAS) have a significant inherent central bias.