Paria Mehrani presents “Multiplicative modulations in hue-selective cells enhance unique hue representation” at ICVS 2019
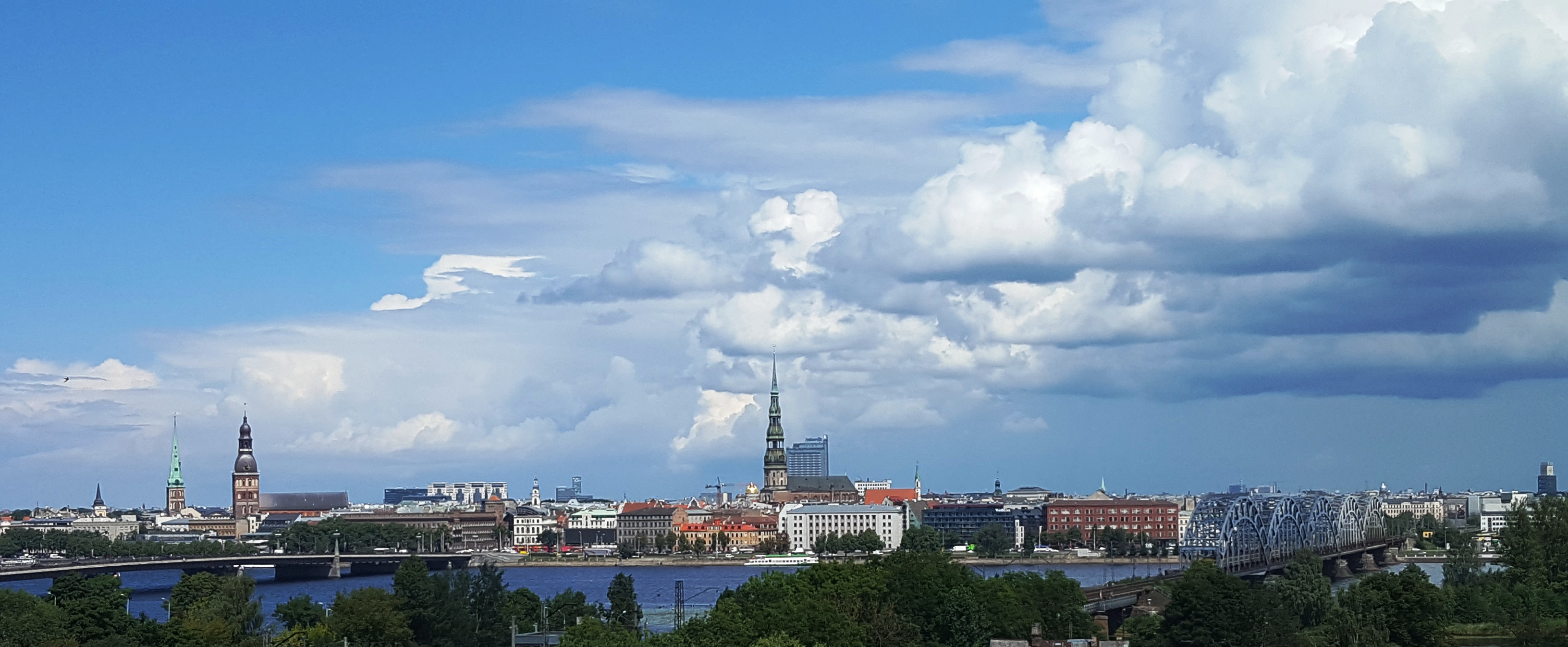
Venue: ICVS 2019, Latvia
Paper:Multiplicative modulations in hue-selective cells enhance unique hue representation
Abstract:
Which region in the brain represents unique hues is a matter of debate among color vision researchers [1, 2]. Here, we introduce a hierarchical model inspired by neural mechanisms in the visual system for local hue representation and with computational simulations suggest that neurons in V4 and beyond have the capacity to encode unique hues. Our model builds a network of single-opponent color and hue-selective neurons that successfully encodes local hue. Our network differs from that of [3, 4] as it models neurons in each of LGN, V1, V2, and V4 areas and explicitly reveals how the contributions of each visual cortical area participating in the process can lead to a hue representation. Our network receives cone responses as input and achieves local hue encoding through gradually increasing nonlinearities in terms of cone inputs as observed by [5]. Specifically, single-opponent color LGN responses are obtained by linearly combining cone activation. In V1, half-wave rectification keeps tunings of these cells similar to those of LGN neurons as suggested by [6], while V1 cells become nonlinear in terms of cone inputs. In V2, in addition to single-opponent color cells, we propose employing neurons with multiplicative modulations. De Valois et al. [3] suggested that additive or subtractive modulation of cone-opponent cells with S-opponent cell responses rotates the cone-opponent axes to redgreen and blue-yellow directions. Here, we achieved this rotation with multiplicative modulations of V1 L- and M-opponent cell activations with V1 S-opponent neuron responses. Multiplicative modulations of nonlinear V1 responses increase nonlinearities in cone input and allow for mixing of color channels. Furthermore, unlike additive and subtractive modulations with no notable impact on tuning bandwidths, multiplicative modulations decrease V2 tuning bandwidths. Finally, V4 responses are computed by linearly combining V2 activations with weights determined according to tuning peak distances of V2 cells to the desired V4 neuron tuning peak. Our experimental results indicate that multiplicatively modulated V2 cells play an important role in the representation of hues along intermediate directions in the MacLeodBoyton diagram [7]. Similarly, these neurons have substantial input weights compared to singleopponent V2 cells to V4 neurons selective to unique green and blue hues. Moreover, we observed a gradual decrease in distance of tuning peaks to unique hue angles reported by [8] from our model LGN cells to V4 neurons. Our simulation results demonstrate that responses of our network neurons resemble those of biological color cells.