Paria Mehrani presents “Learning a model of shape selectivity in V4 cells reveals shape encoding mechanisms in the brain” at VSS 2021
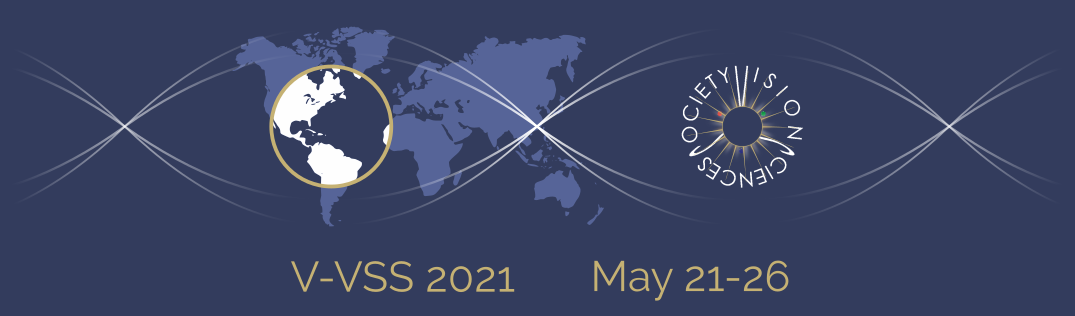
Venue: VSS 2021, Virtual
Paper:Learning a model of shape selectivity in V4 cells reveals shape encoding mechanisms in the brain
Abstract:
The mechanisms of local shape information transformation from V1 to more abstract representations in IT are unknown. Studying the selectivities in intermediate stages of transformation suggest plausible mechanisms. For example, Pasupathy and Conner [1] studied Macaque V4 responses to convexities and concavities. They found that these neurons are selective to boundary configurations at a specific position in the stimulus, for example, a convexity adjacent to a concavity. Although such investigations reveal intermediate shape representations in the brain, they often do not suffice in capturing complex and long-range interactions within the receptive field due to imposing priors on tunings, e.g., fitting a single Gaussian to neuron responses. Here, we propose a learning-based approach that eliminates the need for such strong priors. Specifically, we investigate shape representation in Macaque V4 cells and formulate shape tuning as a sparse-coding problem according to previous findings of V4 neurons[1]. We emphasize that our goal is not to find a mapping from the stimulus to V4 responses but to study how V4 neurons combine responses of curvature-selective V2 cells to achieve their reported part-based selectivities. To this end, our proposed model takes responses of simulated curvature-selective V2 cells as input by combining two previously introduced hierarchical models [2,3]. With simulated curvature signal as input, our algorithm learns a sparse mapping to V4 responses that reveals each Macaque V4 cell’s tuning and the mechanism by which the tuning is achieved. Our model captures sophisticated interactions within the receptive field from neuron responses. Our results on V4 shape representations confirm long-range interactions between components of a larger shape, providing a better understanding of shape encoding mechanisms in the brain.