Saliency
Visual Saliency
Among the very first implementations of a saliency model were the Culhane & Tsotsos 1992 efforts. A couple of decades later, the AIM (Attention via Information Maximization) appeared and has become a standard benchmark in the field (Bruce & Tsotsos 2005, 2009).
Extensions to binocular camera data, to robotic visual search, to incorporating task instructions (STAR-FCT) and more have also appeared. More recently, an eye fixation controller, STAR-FC, has been demonstrated to have performance virtually identical to human performance. See also the STAR-FC project under Visual Architectures, as well as other related projects in the current category.
Publications
Tsotsos, J. K., Kotseruba, I., & Wloka, C. (2019). Early Salient Region Selection Does Not Drive Rapid Visual Categorization. arXiv preprint arXiv:1901.04908.
Berga D, Wloka C, Tsotsos JK and Lassonde School of Engineering – York University. Modeling task influences for saccade sequence and visual relevance prediction. F1000Research 2019, 8:820 (poster) (https://doi.org/10.7490/f1000research.1116873.1)
Wloka, C., Kunić, T., Kotseruba, I., Fahimi, R., Frosst, N., Bruce, N. D., & Tsotsos, J. K. (2018). SMILER: Saliency Model Implementation Library for Experimental Research, arXiv:1812.08848
Bruce, N., Wloka, C., Tsotsos, J.K., On Computational Modeling of Visual Saliency: Understanding What’s Right and What’s Left, Special Issue on Computational Models of Visual Attention, Vision Research, Volume 116, Part B, November 2015, Pages 95-112
Bylinskii, Z., DeGennaro, E., Rajalingham, R., Ruda, H., Zhang, J., Tsotsos, J.K., Towards the quantitative evaluation of visual attention models, Special Issue on Computational Models of Visual Attention, Vision Research, Volume 116, Part B, November 2015, Pages 258-268
Rasouli, A., & Tsotsos, J. K. (2014, May). Visual saliency improves autonomous visual search. In 2014 Canadian Conference on Computer and Robot Vision (pp. 111-118). IEEE.
Andreopoulos, A., & Tsotsos, J. K. (2011). On sensor bias in experimental methods for comparing interest-point, saliency, and recognition algorithms. IEEE transactions on pattern analysis and machine intelligence, 34(1), 110-126.
Bruce, N. D., & Tsotsos, J. K. (2009). Saliency, attention, and visual search: An information theoretic approach. Journal of vision, 9(3), 5-5.
Bruce, N. D., & Tsotsos, J. K. (2008, May). Spatiotemporal saliency: Towards a hierarchical representation of visual saliency. In International Workshop on Attention in Cognitive Systems (pp. 98-111). Springer, Berlin, Heidelberg.
Bruce, N., & Tsotsos, J. (2005). Saliency based on information maximization. In Advances in neural information processing systems (pp. 155-162).
Culhane, S., Tsotsos, J.K., A Prototype for Data-Driven Visual Attention, 11th ICPR, The Hague, August 1992, pp. 36 – 40.
Culhane, S. and Tsotsos, J. An Attentional Prototype for Early Vision, Proceedings of the Second European Conference on Computer Vision, Santa Margherita Ligure, Italy, May 1992, G. Sandini (Ed.), LNCS-Series Vol. 588, Springer Verlag, pages 551-560.
SMILER
The Saliency Model Implementation Library for Experimental Research (SMILER) provides a common, standardized API for saliency model execution, drastically reducing the effort required to run and test saliency model behaviour while also improving consistency and reproducibility in saliency research. By reducing the effort required to run saliency models, we aim to enable new, more robust tests of saliency model behaviour in order to determine new paths forward for computational modeling of saliency. In particular,
Key results
– In the BMVC paper we find that no model
Publications
Calden Wloka, Toni Kunić, Iuliia Kotseruba, Ramin Fahimi, Nicholas Frosst, Neil D. B. Bruce, and John K. Tsotsos (2018) SMILER: Saliency Model Implementation Library for Experimental Research. arXiv preprint.
Iuliia Kotseruba, Calden Wloka, Amir Rasouli, John K. Tsotsos (2019) Do Saliency Models Detect Odd-One-Out Targets? New Datasets and Evaluations. British Machine Vision Conference (BMVC)
Code is available from https://github.com/TsotsosLab/SMILER, and worked examples can be found at http://www.cse.yorku.ca/~calden/projects/smiler.html
Do Saliency Models Detect Odd-One-Out Targets?
Current fixation prediction benchmarks are nearing saturation but the problem of predicting human gaze is far from being solved. In particular, we show that both classical (theory-driven) and modern (data-driven) saliency algorithms fail to capture fundamental properties of human attention. We demonstrate both on synthetic patterns frequently used in psychophysics research and on a new dataset of odd-one-out images gathered “in the wild” that saliency models are not able to detect targets that significantly differ from the surrounding in color, orientation or size.
Key Results
A systematic investigation of 12 classical and 8 deep learning models on two new datasets: P3 (psychophysical patterns) and O3 (naturalistic odd-one-out images); classical models perform better on synthetic patterns but deep models perform better on natural images; deep models have highly inconsistent performance on similar targets; color targets are easier for all models to find, while size and orientation are more difficult; none of the models can capture all three types of features considered.


Publications
I. Kotseruba, C. Wloka, A. Rasouli, J.K. Tsotsos, “Do Saliency Models Detect Odd-One-Out Targets? New Datasets and Evaluations”, British Machine Vision Conference (BMVC), 2019.
STAR-FC
The Selective Tuning Attentive Reference Fixation Control (STAR-FC) model provides a dynamic representation of attentional priority as it predicts an explicit saccadic sequence over visual input, moving beyond a static saliency representation for fixation prediction. STAR-FC provides a link between saliency and higher level models of visual cognition, and serves as a useful module for a broader active vision architecture.
equence over visual input, moving beyond a static saliency representation for fixation prediction. STAR-FC provides a link between saliency and higher level models of visual cognition, and serves as a useful module for a broader active vision architecture.
Key results
– High fidelity reproduction of the first five fixations over an image
– Qualitatively more natural sequence structure for long duration saccade sequences
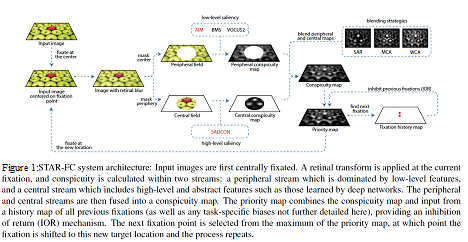
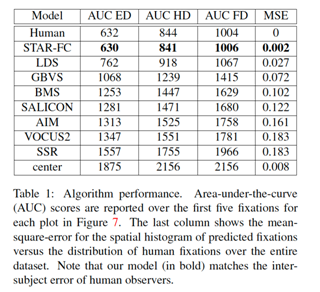
Publications
Calden Wloka, Iuliia Kotseruba, and John K. Tsotsos (2018) Active Fixation Control to Predict Saccade Sequences. Proc. of Conference on Computer Vision and Pattern Recognition (CVPR)
John K. Tsotsos, Iuliia Kotseruba, and Calden Wloka (2016) A Focus on Selection for Fixation. Journal of Eye Movement Research 9(5):2,1-34
Code is available from https://github.com/TsotsosLab/STAR-FC
For more information visit:
http://www.cse.yorku.ca/~calden/projects/fixation_control.html
STAR-FCT
In this work we propose an architecture to enable tuning of attentional processing mechanisms to adapt vision given specific task instructions. We will extend to the Selective Tuning Attentive Reference Fixation Controller (STAR-FC) by incorporating:
– Long-Term Memory (LTM) for Symbolic and Visual Representations
– Task Working Memory (tWM) for Location bias with respect task specification
– Visual Task Executive (vTE) to Fetch methods from LTM to Hierarchy & tWM
We hope to provide low- and high-level feature basis for the visual memory representations, to define a hierarchical lexico-semantic memory explaining the task specification, determine influences of task over fixations (including memory, covert and overt attention).
This work is led by our collaborator, Dr. David Berga, Computer Vision Center, Autonomous University of Barcelona, Spain.
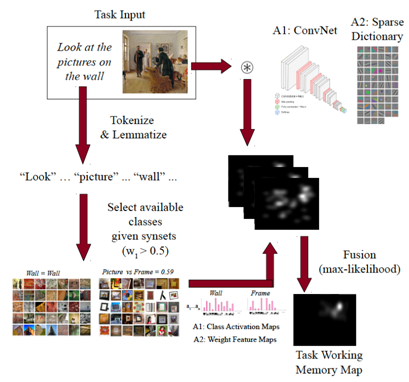
Publications
Berga D, Wloka C, Tsotsos JK and Lassonde School of Engineering – York University. Modeling task influences for saccade sequence and visual relevance prediction. F1000Research 2019, 8:820 (poster) (https://doi.org/10.7490/f1000research.1116873.1)