Selective Tuning
STNet: Selective Tuning of Convolutional Networks for Object Localization
Visual attention modeling has recently gained momentum in developing visual hierarchies provided by Convolutional Neural Networks. Despite recent successes of feedforward processing on the abstraction of concepts form raw images, the inherent nature of feedback processing has remained computationally controversial. Inspired by the computational models of covert visual attention, we propose the Selective Tuning of Convolutional Networks (STNet). It is composed of both streams of Bottom-Up and Top-Down information processing to selectively tune the visual representation of Convolutional networks. We experimentally evaluate the performance of STNet for the weakly-supervised localization task on the ImageNet benchmark dataset. We demonstrate that STNet not only successfully surpasses the state-of-the-art results but also generates attention-driven class hypothesis maps.
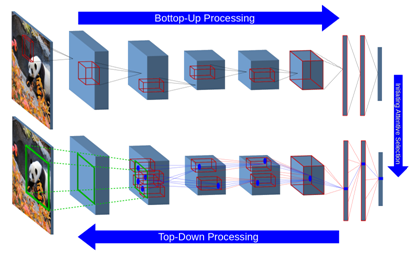
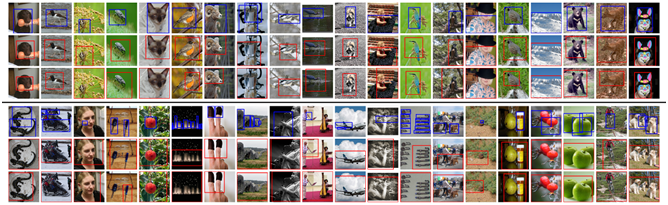
Publications
Biparva, John Tsotsos, STNet: Selective Tuning of Convolutional Networks for Object Localization, The IEEE International Conference on Computer Vision (ICCV) Workshops, 2017, pp. 2715-2723
Code is available from https://gitlab.nvision.eecs.yorku.ca/shared/stnetdockerscript
Priming Neural Networks
Visual priming is known to affect the human visual system to allow detection of scene elements, even those that may have been near unnoticeable before, such as the presence of camouflaged animals. This process has been shown to be an effect of top-down signaling in the visual system triggered by the said cue. In this paper, we propose a mechanism to mimic the process of priming in the context of object detection and segmentation. We view priming as having a modulatory, cue dependent effect on layers of features within a network. Our results show how such a process can be complementary to, and at times more effective than simple post-processing applied to the output of the network, notably so in cases where the object is hard to detect such as in severe noise. Moreover, we find the effects of priming are sometimes stronger when early visual layers are affected. Overall, our experiments confirm that top-down signals can go a long way in improving object detection and segmentation.
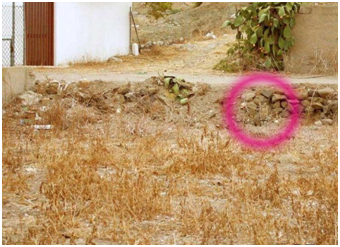
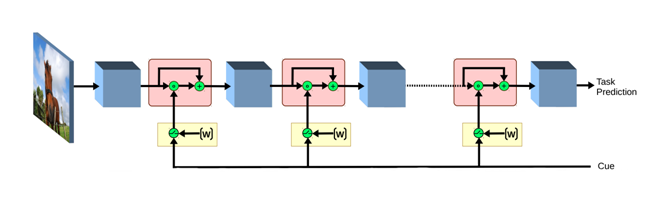
Publications
Rosenfeld, Amir and Biparva, Mahdi and Tsotsos, John K., Priming Neural Networks, The IEEE Conference on Computer Vision and Pattern Recognition (CVPR) Workshops, June, 2018
STAR-FC
The Selective Tuning Attentive Reference Fixation Control (STAR-FC) model provides a dynamic representation of attentional priority as it predicts an explicit saccadic sequence over visual input, moving beyond a static saliency representation for fixation prediction. STAR-FC provides a link between saliency and higher level models of visual cognition, and serves as a useful module for a broader active vision architecture.
equence over visual input, moving beyond a static saliency representation for fixation prediction. STAR-FC provides a link between saliency and higher level models of visual cognition, and serves as a useful module for a broader active vision architecture.
Key results
– High fidelity reproduction of the first five fixations over an image
– Qualitatively more natural sequence structure for long duration saccade sequences
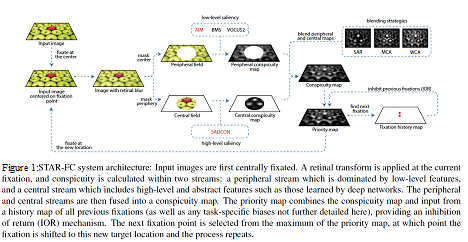
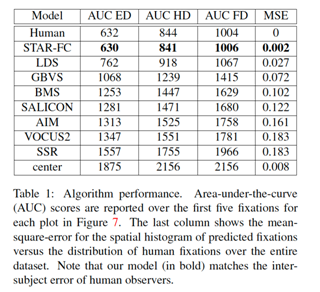
Publications
Calden Wloka, Iuliia Kotseruba, and John K. Tsotsos (2018) Active Fixation Control to Predict Saccade Sequences. Proc. of Conference on Computer Vision and Pattern Recognition (CVPR)
John K. Tsotsos, Iuliia Kotseruba, and Calden Wloka (2016) A Focus on Selection for Fixation. Journal of Eye Movement Research 9(5):2,1-34
Code is available from https://github.com/TsotsosLab/STAR-FC
For more information visit:
http://www.cse.yorku.ca/~calden/projects/fixation_control.html
STAR-FCT
In this work we propose an architecture to enable tuning of attentional processing mechanisms to adapt vision given specific task instructions. We will extend to the Selective Tuning Attentive Reference Fixation Controller (STAR-FC) by incorporating:
– Long-Term Memory (LTM) for Symbolic and Visual Representations
– Task Working Memory (tWM) for Location bias with respect task specification
– Visual Task Executive (vTE) to Fetch methods from LTM to Hierarchy & tWM
We hope to provide low- and high-level feature basis for the visual memory representations, to define a hierarchical lexico-semantic memory explaining the task specification, determine influences of task over fixations (including memory, covert and overt attention).
This work is led by our collaborator, Dr. David Berga, Computer Vision Center, Autonomous University of Barcelona, Spain.
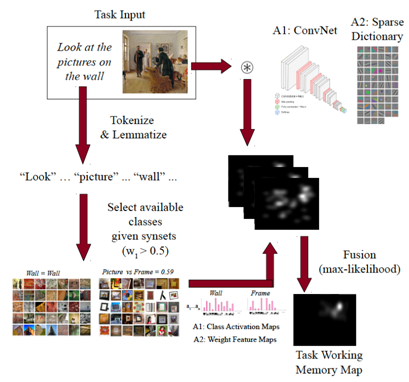
Publications
Berga D, Wloka C, Tsotsos JK and Lassonde School of Engineering – York University. Modeling task influences for saccade sequence and visual relevance prediction. F1000Research 2019, 8:820 (poster) (https://doi.org/10.7490/f1000research.1116873.1)
Attentional surround suppression: Location-based and feature-based approaches
The Selective Tuning model has proposed that the focus of attention, either spatial location or a visual feature, is accompanied by a suppressive surround to contrast the attended and unattended information. In the present project, I investigate the factors that manipulate the properties of a location-based suppressive surround, and the interaction between the location-based and feature-based surround suppression mechanisms. I find that stimulus eccentricity, correlated with neurons’ RF sizes, modulates the size of the suppressive surround and the profile of the suppressive surround is influenced by both spatial proximity and feature similarity of visual items, suggesting an interplay between the two suppressive mechanisms. In addition, the present project provides behavioral and neurophysiological evidence for feature-based surround suppression in motion processing.
This work is in collaboration with Dr. Mazyar Fallah, York University, Toronto, Canada.
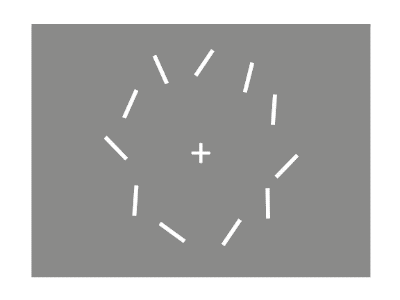
Publications
Yoo, S-. A., Tsotsos, J. K., & Fallah, M. (2018). The attentional suppressive surround: Eccentricity, location-based and feature-based effects and interactions. Frontiers in Neuroscience. 12:710. doi: 10.3389/fnins.2018.00710
Yoo, S-. A., Martinez-Trujillo, J., Treue, S., Tsotsos, J. K., & Fallah, M. (2019). Feature-based surround suppression in motion processing. (it will be available in biorxiv soon).
The roles of top-down feedback processing in visual perception
People can perform simple visual tasks very rapidly and accurately, suggesting the sufficiency of feed-forward visual processing for these tasks. However, more complex visual tasks may require top-down feedback processing to access fine-grained information available at earlier processing levels in the visual hierarchy, thus, additional processing time is needed for this downward traversal. The goal of this project is measuring processing time taken to complete different visual recognition tasks (detection and localization of targets) in simple or complex visual scenes. Task performance in simple scenes becomes asymptotic within a short period of time. On the other hand, task performance in cluttered scenes improves when additional processing time is allowed, indicating that slow, time-consuming top-down feedback processing contributes to analyze elements in complex visual scenes.
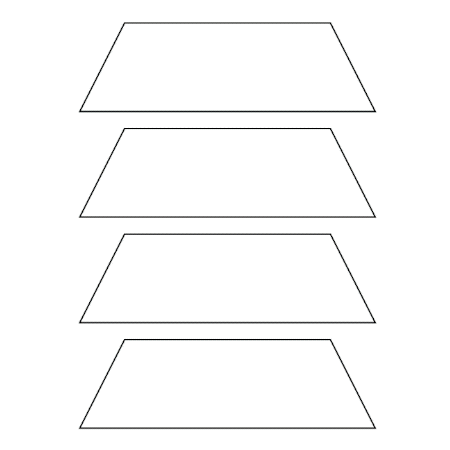
Publications
Yoo, S-. A., Tsotsos, J. K., & Fallah, M. (under revision). Feed-forward visual processing suffices for coarse localization but precise localization in a complex context needs feedback processing.
Adaptive Representations
Easily use learned representations for new tasks. An alternative to transfer learning that fully eliminates catastrophic forgetting, while requiring significantly less parameters and converges faster.
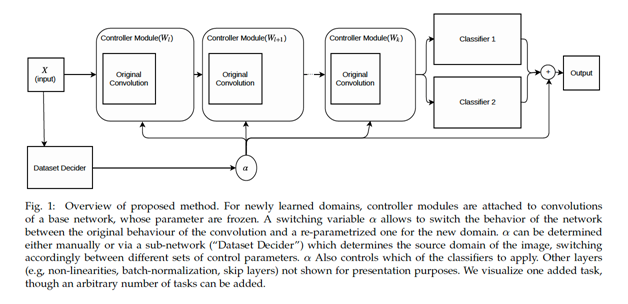
Publication
Rosenfeld, A., & Tsotsos, J. K. (2018). Incremental learning through deep adaptation. IEEE transactions on pattern analysis and machine intelligence.
Shortcomings of Deep Learning vs the Human Visual System
Find where current computer-vision severely lacks w.r.t human vision in order to improve the former. In short: revealing a family of errors made by state-of-the-art object detectors, induced by simple copying and pasting of learned objects in arbitrary image locations. It is suggested that these errors stem from the lack of a mechanism for inhibiting interfering signals within the receptive field of the detector.
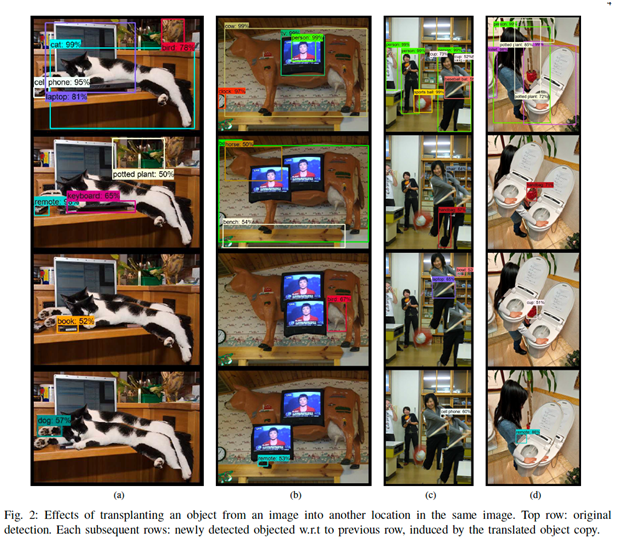
Link to the youtube video
Publication
Rosenfeld, A., Zemel, R., & Tsotsos, J. K. (2018). The elephant in the room. arXiv preprint arXiv:1808.03305.
Selective Tuning of Convolutional Network for Affine Motion Pattern Classification and Localization
Tsotsos et al. (2004) present the first motion-processing model to include a multi-level decomposition with local spatial-derivatives of velocity and demonstrated localization of motion patterns using selective tuning. Later, Vintila and Tsotsos (2007) present motion detection and estimation for attending to visual motion using orientation tensor formalism method. Velocity field is extracted using a model with 9 quadrature filters. Here we try to develop a learning framework for these motions with the same goal: Classify affine motion patterns using convolutional network and localize motion patterns using selective tuning algorithm. Evaluate performance of different network architectures on these tasks.
Key Results
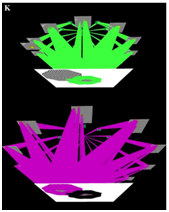
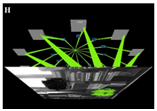
Publications
Vintila, F., Tsotsos, J.K., Motion Estimation using a General Purpose Neural Network Simulator for Visual Attention, IEEE Workshop: Applications of Computer Vision, Austin, Texas, Feb. 21-22, 2007.
Tsotsos, J.K., Liu, Y., Martinez-Trujillo, J., Pomplun, M., Simine, E., Zhou, K., Attending to Visual Motion, Computer Vision and Image Understanding 100(1-2), p 3 – 40, Oct. 2005.
Attentive Compact Neural Representation
Deep neural networks have evolved to become power demanding and consequently difficult to apply to small-size mobile platforms. Network parameter reduction methods have been introduced to systematically deal with the computational and memory complexity of deep networks. We propose to examine the ability of attentive connection pruning to deal with redundancy reduction in neural networks as a contribution to the reduction of computational demand. In this chapter, we describe a Top-Down attention mechanism that is added to a Bottom-Up feedforward network to select important connections and subsequently prune redundant ones at all parametric layers. Our method not only introduces a novel hierarchical selection mechanism as the basis of pruning but also remains competitive with previous ad hoc methods in experimental evaluation. We conduct experiments using different network architectures on popular benchmark datasets to show high compression rate is achievable with negligible loss of accuracy.
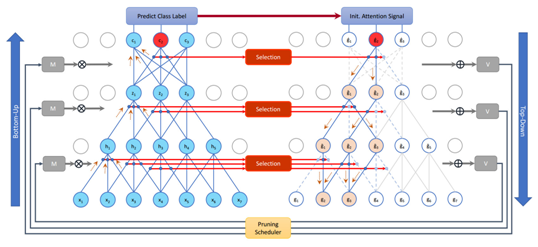
Publications
Biparva, M., Top-Down Selection in Convolutional Neural Networks, Doctoral Dissertation, Dept. of Electrical Engineering and Computer Science, York University, September 2019.
Contextual Interference Reduction by Focusing on Attentional Traces
The issue of the contextual interference with the foreground target objects is one of the main shortcomings of the hierarchical feature representations such as convolutional neural networks. Due to the dense hierarchical parametrization of convolutional neural networks and the utilization of convolution and sub-sampling layers in the feedforward manner, foreground and background representations are inevitably mixed up and visual confusion is eminent. A systematic approach to shift learned neural representations from the emphasis on the contextual regions to the foreground target objects can help achieve a higher degree of representation disentanglement. We propose a selective fine-tuning approach for neural networks using a unified bottom-up and top-down framework. A gating mechanism of hidden activities imposed by Top-Down selection mechanisms is defined in the iterative feedforward pass. An attention-augmented loss function is introduced using which the network parameters are fine-tuned for a number of iterations. The fine-tuning using the iterative pass helps the network to reduce the reliance on the contextual representation throughout the visual hierarchy. Therefore, the label prediction relies more on the target object representation and consequently achieve a higher degree of robustness to the background changes.
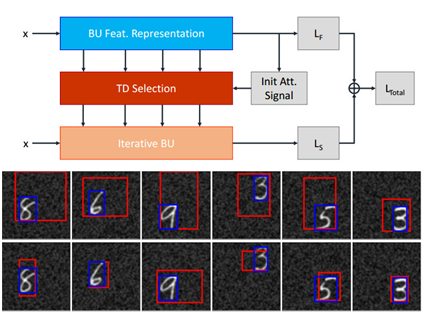
Publications
Biparva, M., Top-Down Selection in Convolutional Neural Networks, Doctoral Dissertation, Dept. of Electrical Engineering and Computer Science, York University, September 2019.
Neurophysiology of Attention
Ongoing experimental research on human visual attention is conducted with our collaborators at the Otto-von-Guericke University of Magdeburg, Germany, led by Jens-Max Hopf. They use MEG (Magnetoencephalography) imaging primarily to investigate many of the predictions of the Selective Tuning model. A nice presentation of the work done up to 2018 appears in a lecture by Hopf here.
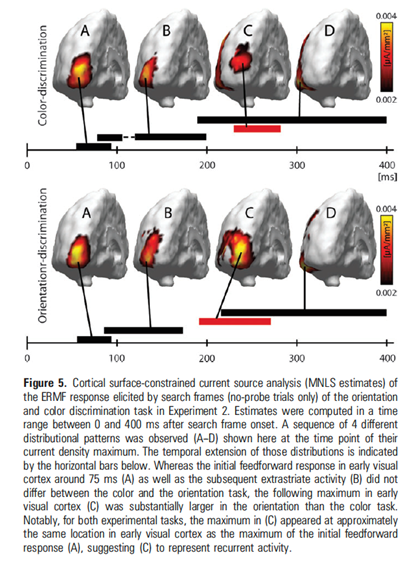
Publications
Bartsch, M.V, Loewe, K., Merkel, C., Heinze, H.-J., Schoenfeld, M. A., Tsotsos, J.K., Hopf, J.-M., Attention to color sharpens neural population tuning via feedback processing in the human visual cortex hierarchy, J. Neuroscience 25 October 2017, 37 (43) 10346-10357.
Boehler, C.N., Tsotsos, J.K., Schoenfeld, M., Heinze, H.-J., Hopf, J.-M., Neural mechanisms of surround attenuation and distractor competition in visual search, The Journal of Neuroscience, 31(14), p5213–5224, April 6, 2011.
Hopf, J.-M., Boehler, N., Schoenfeld, M., Heinze, H.-J., Tsotsos, J.K., The spatial profile of the focus of attention in visual search: Insights from MEG recordings, Vision Research 50(14), p.1312-1320, 2010.
Boehler, C.N., Tsotsos, J.K., Schoenfeld, M., Heinze, H.-J., Hopf, J.-M., The center-surround profile of the focus of attention arises from recurrent processing in visual cortex, Cerebral Cortex, 19:982-991 , 2009; (published online on August 28, 2008, doi:10.1093/cercor/bhn139).
Hopf, J.-M., Boehler C.N., Luck S.J., Tsotsos, J.K., Heinze, H.-J., Schoenfeld M.A., Direct neurophysiological evidence for spatial suppression surrounding the focus of attention in vision, Proceedings of the National Academy of Sciences, Biological Track,103(4), p1053-8. (Epub 2006 Jan 12), 2006 Jan 24, 2006.
Psychophysics of Attention
We have ongoing efforts to test a variety of predictions of the Selective Tuning model in human perception. The predictions are all documented in the basic papers on the model, which can be found under the Selective Tuning project link.
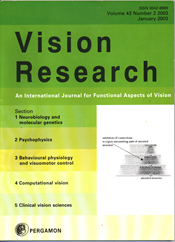
Publications
Audrey M. B. Wong-Kee-You, John K. Tsotsos, Scott A. Adler; Development of spatial suppression surrounding the focus of visual attention. Journal of Vision 2019;19(7):9. doi: 10.1167/19.7.9.
Audrey M. B. Wong-Kee-You, John K. Tsotsos, Scott A. Adler; Development of spatial suppression surrounding the focus of visual attention. Journal of Vision 2019;19(7):9. doi: 10.1167/19.7.9.
Bruce ND, Tsotsos JK (2011) Visual representation determines search difficulty: explaining visual search asymmetries. Front. Comput. Neurosci. 5:33. doi: 10.3389/fncom.2011.00033, Received: 22 February 2011; Accepted: 24 June 2011; Published online: 13 July 2011.
Loach, D., Frischen, A., Bruce, N., Tsotsos, J.K., An attentional mechanism for selecting appropriate actions afforded by graspable objects, Psychological Science 19(12), p 1253-1257, 2008. Published Online: Dec 15 2008
Tombu, M., Tsotsos, J.K., Attending to Orientation Results in an Inhibitory Surround in Orientation Space, Perception & Psychophysics 70 (1), p30-35, 2008.
Loach, D., Botella, J., Privado, J., Tsotsos, J.K., Priming and Intrusion Errors in RSVP Streams with Two Response Dimensions, Psychological Research 72(3), p281-288, 2008.
Cutzu, F., Tsotsos, J.K., The selective tuning model of visual attention: Testing the predictions arising from the inhibitory surround mechanism, Vision Research, 43(2), p205-19, Jan. 2003
STAR – The Selective Tuning Attentive Reference Cognitive Architecture
What are the computational tasks that an executive controller for visual attention must solve? This question is posed in the context of the Selective Tuning model of attention. The range of required computations go beyond top-down bias signals or region-of-interest determinations, and must deal with overt and covert fixations, process timing and synchronization, information routing, memory, matching control to task, spatial localization, priming, and coordination of bottom-up with top-down information. During task execution, results must be monitored to ensure the expected results. This description includes the kinds of elements that are common in the control of any kind of complex machine or system. We seek a mechanistic integration of the above, in other words, algorithms that accomplish control. Such algorithms operate on representations, transforming a representation of one kind into another, which then forms the input to yet another algorithm. Cognitive Programs (CPs) are hypothesized to capture exactly such representational transformations via stepwise sequences of operations. CPs, an updated and modernized offspring of Ullman’s Visual Routines, impose an algorithmic structure to the set of attentional functions and play a role in the overall shaping of attentional modulation of the visual system so that it provides its best performance. This requires that we consider the visual system as a dynamic, yet general-purpose processor tuned to the task and input of the moment. This differs dramatically from the almost universal cognitive and computational views, which regard vision as a passively observing module to which simple questions about percepts can be posed, regardless of task. Differing from Visual Routines, CPs explicitly involve the critical elements of Visual Task Executive, Visual Attention Executive, and Visual Working Memory. Cognitive Programs provide the software that directs the actions of the Selective Tuning model of visual attention. The STAR architecture ties all of these elements together.
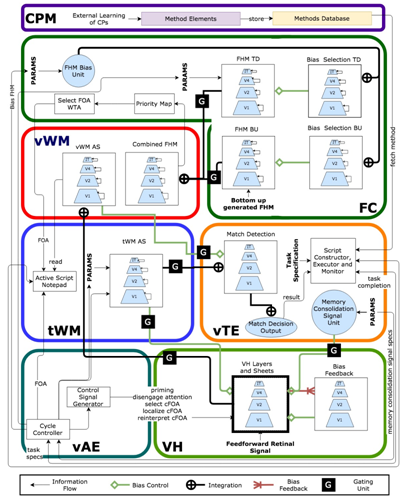
Publications
Abid, O., Cognitive Programs Memory – A framework for integrating executive control in STAR, M.Sc. Thesis, Dept. of Electrical Engineering and Computer Science, York University, December 12, 2018.
Rosenfeld, A., Tsotsos, J.K., Bridging Cognitive Programs and Machine Learning, arXiv Preprint arXiv:1802.06091, February 2018.
Kunic, T., Cognitive Program Compiler, M.Sc. Thesis, Dept. of Electrical Engineering and Computer Science, York University, April 11, 2017.
Tsotsos JK, Kruijne W (2014). Cognitive programs: Software for attention’s executive. Frontiers in Psychology: Cognition 5:1260. doi: 10.3389/fpsyg.2014.01260 (Special Issue on Theories of Visual Attention – linking cognition, neuropsychology, and neurophysiology, edited by S. Kyllingsbæk, C. Bundesen, S. Vangkilde)
Tsotsos JK. Cognitive programs: towards an executive controller for visual attention. F1000Posters 2013, 4:355 (poster)