Spatial Cognition
Totally-Looks Like: Totally Looks Like-How Humans Compare, Compared to Machines
Perceptual judgment of image similarity by humans relies on rich internal representations ranging from low-level features to high-level concepts, scene properties and even cultural associations. Existing methods and datasets attempting to explain perceived similarity use stimuli which arguably do not cover the full breadth of factors that affect human similarity judgments, even those geared toward this goal. We introduce a new dataset and show that current state-of-the-art techniques do not suffice.
Dataset with 6016 image-pairs from the wild, shedding light upon a rich and diverse set of criteria employed by human beings. We conduct experiments to try to reproduce the pairings via features extracted from state- of-the-art deep convolutional neural networks, as well as additional human experiments to verify the consistency of the collected data.
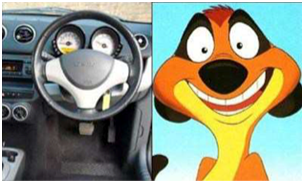
Publications
Rosenfeld, Amir, Markus D. Solbach, and John K. Tsotsos. “Totally looks like-how humans compare, compared to machines.” Proceedings of the IEEE Conference on Computer Vision and Pattern Recognition Workshops. 2018.
Rosenfeld, Amir, Markus Solbach, and John Tsotsos. “Totally-Looks-Like: A Dataset and Benchmark of Semantic Image Similarity.” Journal of Vision 18.10 (2018): 136-136.
Active Observers in a 3D World: The Same-Different Task
There are many examples where a robot that can act as an active observer would be useful. In this project, we focus on the perception-feedback component of the active visual processing pipeline – We want to understand how human subjects use multiple views to recognize objects. To limit the scope of the problem, we study how humans might solve a variation of the popular Same-Different Task and assert that this is a basic perceptual ability. We wish to discover the underlying strategies that are used by humans to solve the 3D Same-Different Taskas active observers. We introduced the 3D Same-Different Task for active observers. Created a novel set of 3D objects with known complexity. We established that the orientation between the two presented objects has a large influence on the subject’s performance – both qualitatively and quantitatively.
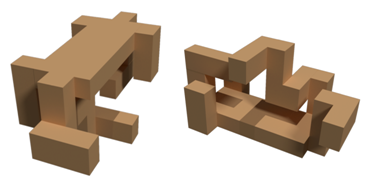
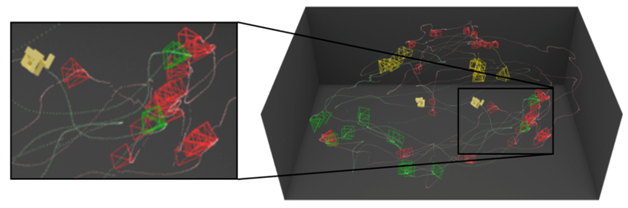
Publications
Tsotsos, John K., et al. “Visual attention and its intimate links to spatial cognition.” Cognitive processing 19.1 (2018): 121-130.
Pedestrian behavior understanding
The goal of this project is to observe and understand pedestrian actions at the time of crossing, and identify the factors that influence the way pedestrians make crossing decision. We intend to incorporate these factors into predictive models in order to improve prediction of pedestrian behavior.
Key Results
We found that join attention, i.e. when pedestrians make eye contact with drivers, is a strong indicator of crossing intention. We identified numerous factors, such as demographics, street structure, driver behavior, that impact pedestrian crossing behavior. The way pedestrians communicate their intention and the meaning of communication signals also were explored. Our initial experimentation suggests that including such information in practical applications can improve prediction of pedestrian behavior.
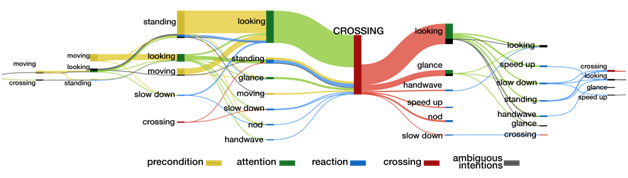
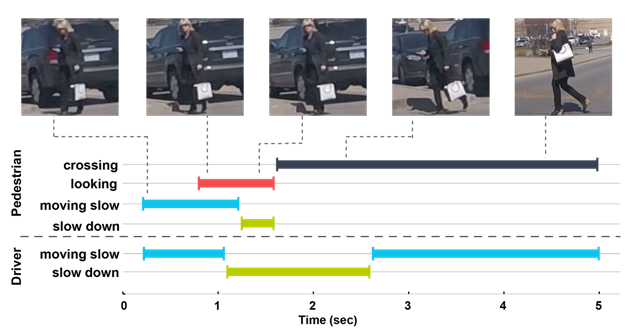
Publications
A. Rasouli and J. K. Tsotsos, “Autonomous vehicles that interact with pedestrians: A survey of theory and practice,” IEEE Transactions on Intelligent Transportation Systems, 2019.
A. Rasouli, I. Kotseruba, and J. K. Tsotsos, “Agreeing to cross: How drivers and pedestrians communicate,” In Proc. Intelligent Vehicles Symposium (IV), 2017, pp. 264–269.
A. Rasouli, I. Kotseruba, and J. K. Tsotsos,“Are they going to cross? a benchmark dataset and baseline for pedestrian crosswalk behavior,” In Proc. International Conference on Computer Vision (ICCV) Workshop, 2017, pp. 206–213.
A. Rasouli, I. Kotseruba, and J. K. Tsotsos, “Towards Social Autonomous Vehicles: Understanding Pedestrian-Driver Interactions,” In Proc. International Conference on Intelligent Transportation Systems (ITSC), pp. 729-734, 2018.
Pedestrian intention estimation
The objective of this project is to develop methods to predict underlying intention of pedestrians on the road. Understanding the intention helps distinguish between pedestrians that will potentially cross the street and the ones that will not do so, e.g. those waiting for a bus. To achieve this objective we want to establish a baseline by asking human participants to observe pedestrians under various conditions and tell us what the the intention of the pedestrians were. We want to use this information to train an intention.
Key Results
Humans are very good at estimating the intention of pedestrians. Overall, there is a high degree of agreement among human observers regarding the intentions of people on the road. From a practical perspective, we found that including a mechanism to estimate pedestrian intention in a trajectory prediction framework can improve the results.
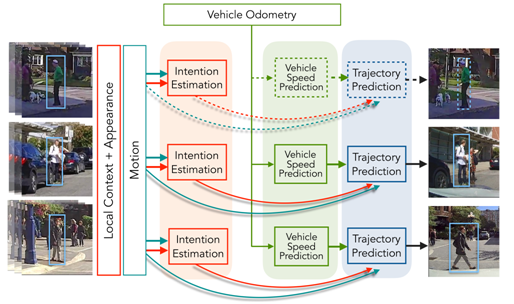
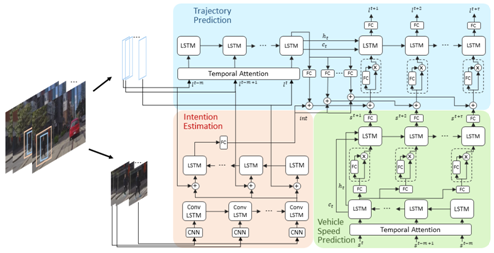
Publications
A. Rasouli, I. Kotseruba, T. Kunic, and J. Tsotsos, “PIE: A Large-Scale Dataset and Models for Pedestrian Intention Estimation and Trajectory Prediction”, ICCV 2019
A. Rasouli, I. Kotseruba, and J. K. Tsotsos, “Pedestrian Action Anticipation using Contextual Feature Fusion in Stacked RNNs”, BMVC 2019.
Active Inductive Inference
The main point of this project is to examine the definition of attention presented in Tsotsos (2011), namely attention is the process by which the brain controls and tunes information processing, and to show how it applies much more broadly than just to visual perception. Visual perception is part of active agents, whether biological or machine, and as such must be effectively integrated into an overall cognitive system that behaves purposely in the real word. This points to the need to expand consideration to include active observers and reasoners. It is not difficult to argue against any purely passive perceptual framework. Passive perception gives up control, and thus any agent that relies on it cannot assert purpose to its behaviour. A passive sensing strategy, no matter how much data are collected, no matter how high the quality of statistics extracted, gives up control over the specific characteristics of what is sensed and at what time and for which purpose. Passive sensing reduces or eliminates the utility of any form of predictive reasoning strategy (hypothesize and test, verification of inductive inferences including Bayesian, etc.). Everyday behaviour relies on sequences of perceptual, decision-making and physical actions selected from a large set of elemental capabilities. It is known that the problem of selecting a sequence of actions to satisfy a goal under resource constraints is known to be NP-hard (Ye and Tsotsos 2001). Humans are remarkably capable regardless. It is a challenge to discover exactly how they are so capable. Inductive inference takes specific premises and makes a broader generalization (conclusion) that is considered probable In a real world (no closed world assumption), conclusions can only be confirmed by an actual test To do so, the system needs to actively tune itself for this test in order to best acquire that data needed.

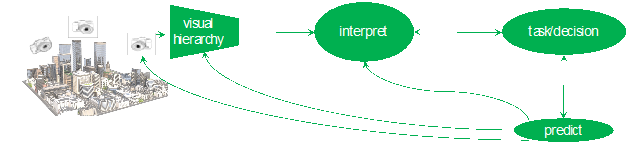
Publications
Tsotsos, J. K., Kotseruba, I., Rasouli, A., & Solbach, M. D. (2018). Visual attention and its intimate links to spatial cognition. Cognitive processing, 19(1), 121-130.
Bajcsy, R., Aloimonos, Y., & Tsotsos, J. K. (2018). Revisiting active perception. Autonomous Robots, 42(2), 177-196.